Fire and Hot Spot Characterization
Background
Beginning with the first generation of satellite fire detection algorithms, the Automated Biomass Burning Algorithm (ABBA) was able to monitor fires with the GOES 4-7 VAS (Visible Infrared Spin Scan Radiometer (VISSR) Atmospheric Sounder) dating back to the early 1980s. Development continued as new GOES Imagers became available starting with GOES-8 through the current generation of GOES Imagers. Since the year 2000, the GOES WF_ABBA has been providing half-hourly diurnal fire products for the Western Hemisphere in near real-time. The software was transitioned to NESDIS operations in 2002. In spite of the relatively coarse resolution of the current GOES Imager (4 km at nadir) and associated limitations in fire detection and characterization, the WF_ABBA user community has greatly expanded over the past five years. Numerous peer-reviewed publications show that user applications include hazards monitoring, climate change research, land-use/land-cover change studies, resource management, biomass burning emissions modeling, diagnostic and prognostic aerosol and trace gas modeling, and policy and decision making. Within the United States biomass burning is a source of aerosols and precursors to ozone formation that must be monitored as mandated by the Clean Air Act with specific PM 2.5 (particulate matter that is 2.5 µm or smaller in size) regulations defined under the 1997 amendment. Biomass burning from both wildfires and agricultural burning remains one of the largest unknowns in source emissions in the U.S. Applications of the GOES WF_ABBA in model data assimilation studies have shown the importance of incorporating real-time diurnal fire products (both fire location and sub-pixel characteristics) in aerosol transport and air quality models to correctly diagnose and predict air pollution.
Product Description
The GOES-R fire product, provides information on the location of the fire pixel; size of the pixel; estimates of instantaneous sub-pixel fire size, temperature, and radiative power; ecosystem type; and a classification flag. In response to a request from the user community, additional information regarding satellite coverage, opaque cloud coverage, block-out zones, and processed regions is also provided. This information, a kind of pixel level metadata and quality indicator, is used in real-time and offline model data assimilation and assessment studies.
Improvements and Benefits
The Fire/Hot Spot Characterization product will make use of both visible and IR spectral bands to locate fires and retrieve sub-pixel fire characteristics. The product will greatly improve upon the currently available Fire Detection product by taking advantage of the higher spatial and temporal resolution which will be available with the GOES-R ABI. Forecasters will be able to use this product to monitor wildfires, and more importantly, rapid changes in individual fires. Forecasters will use this product as part of an arsenal of forecasting tools aimed at helping firefighting efforts.
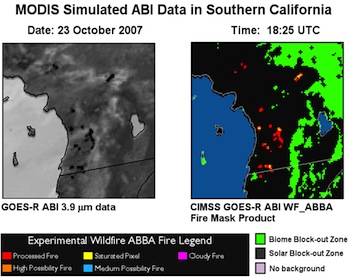
Example of the Fire/Hot Spot Characterization product as derived by the GOES-R Fire/Hot Spot Characterization algorithm using simulated GOES-R ABI 3.9 μm data over Southern California at 1825 UTC on 23 October 2007.
How does it work? - Algorithm
The ABI fire algorithm is a dynamic, multi-spectral, thresholding contextual algorithm that uses the short-wave 0.64 μm (ABI Channel 2, when available during the daytime) and the 3.9 μm and 11.2 μm bands (ABI Channels 7 and 14) to locate fires and retrieve sub-pixel fire characteristics. Channel 15 (12.3 μm) is used along with the aforementioned bands to help identify opaque clouds, but is not required for the algorithm to run. Only Channels 7 and 14 are required for the fires algorithm. The code uses a two-step approach to identify and characterize sub-pixel fires. The first step (known historically as Part I) loops over all pixels and identifies potential fire pixels as well as block-out zones due to solar reflection and select surface types. This initial pass also characterizes possible fire pixels when they meet certain criteria. For each hot pixel the algorithm incorporates ancillary data to screen for false alarms, correct for water vapor attenuation, surface emissivity, solar reflectivity, and semi-transparent clouds. The algorithm utilizes the Dozier technique to calculate sub-pixel estimates of instantaneous fire size and temperature (Dozier, 1981; Matson and Dozier, 1981). Fire Radiative Power (FRP) is also calculated. Fires are treated as a single sub-pixel entity of a certain size, temperature, and radiative power, an approximation that must be made given the fundamental limitations of retrieving sub-pixel properties. The second step (known historically as Part II) loops over all possible fire pixels identified in Part I, additional thresholds are applied, and previous fire detections are used to filter out false alarms.
See the GOES-R ATBD page for all ATBDs.
How are the results compared to existing data? - Calibration and Validation
The ABI active fire validation protocol uses simultaneous active fire observations and verification of burning based on a posteriori burned area maps, and inter-comparison with other validated satellite fire products of higher spatial resolution.
Fire characterization validation is assessed with data from airborne imagers flown by U.S., and potentially international, partners such as the US Forest Service and NASA missions flying the Autonomous Modular Sensor using the Wildfire configuration. Cross-validation of fire characterizations using other similar satellite products has proven limiting [Schroeder et al., 2010] as other products also suffer from the lack of "truth" data. Additionally, such comparisons are limited by differences in resolution and observation geometry.
A more technical validation presentation, (PDF, 16.19 MB) is also available.